For years, studies on strategies have aimed at a sustainable competitive advantage as the ideal strategy for growth and business perpetuation. Innovation has become essential.
Recently, with the advent of AI transforming into a differentiated “workforce”, organizations have begun to reap significant results with its application.
Engineering is not just about numbers and calculations, but also about people, processes, projects, equipment, schedules, and texts. There are uses of AI in text reading, computer vision, bots, and mainly with machine learning for prediction, decision making, classification, among others.
In product engineering, creation, development, and commercialization of products, especially with a high volume of materials, the gain is significant. This article will address some details about the use of AI in product engineering, from research to sales forecasting with the impact of rain on predictive sales models that have changed the way an industry operates and will operate in the future.
Sustainable Competitive Advantage with AI
The evolution of strategic management literature, starting from Porter to Mintzberg, Prahalad, and the blue ocean strategy, makes it possible to envision a strategy that everyone was looking for: how to stay competitive, how to win and find better paths than competitors. In summary, how to achieve a sustainable, permanent competitive advantage.
In an article published by Accenture, in Brazil by Ovanessoff and Plastino (2017), AI was presented as a new factor of production (Figure 1) that could potentially double the economic growth rate of countries (Figure 2).
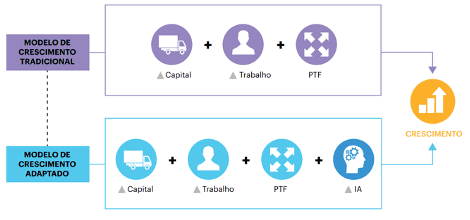
Figure 1 – AI as a New Factor of Production
Source: Ovanessoff and Plastino (2017, p. 9).
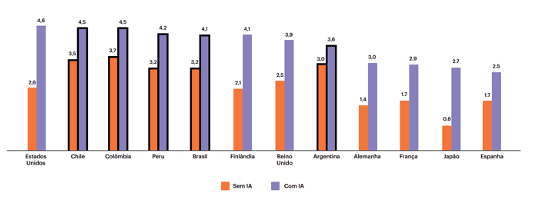
Figure 2 – AI in Economic Growth
Source: Ovanessoff and Plastino (2017, p. 15).
It is possible to achieve competitive advantage with data science / AI. According to Provost and Fawcett (2016, p. 317), data capability and data science are strategic (complementary) assets. If the competition does not have the same asset, with the same quality, or even if they cannot obtain the same value, we have a prerequisite for competitive advantage (PROVOST; FAWCETT, 2016, p. 318).
Once having a competitive advantage with AI, it will be sustainable if the assets cannot be duplicated/copied, or if the techniques used by the data science team are ahead of the competition (PROVOST; FAWCETT, 2016, p. 318).
This view complements that of Barney and Hesterly (2008) on the tool for analyzing sustainable competitive advantage – VRIO. The VRIO acronym refers to the criteria of Valuable, Rare, “Inimitable” and Organizationally Integrated. Valuable, rare, and inimitable refer to data, team, and techniques, but organizationally integrated, at scale, with the organization being able to leverage its results, requires AI maturity. The focus is to be “sustainable”, otherwise, it will be quickly reached by the competition.
The competitive advantage will increase with the company’s maturity in AI / data science. Figure 3 presents the maturity model – Artificial Intelligence Maturity Model from Gartner – AIMM (SICULAR et al., 2020). The results with AI and the competitive advantage grow as maturity increases. Level 4 and 5 generate the integration and connection necessary for the scale of value and the possibility of transforming the organization into a data-driven decision-making vision.
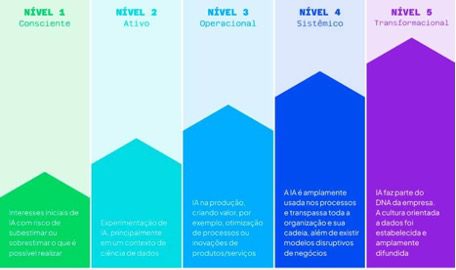
Figure 3 –Artificial Intelligence Maturity Model
Source: Adapted from Gartner AIMM – https://www.gartner.com/en/documents/3982174
In the pursuit of these significant results, AI can bring various gains to organizations, as per table 1, which are obtained differently for each developed use case.
Cost Reduction |
Time Reduction |
Decision Making |
Productivity Increase |
New Business / Revenue Increase |
Process / Performance Improvement |
Risk Reduction |
Greater Convenience / Experience |
Scalability |
Brand Valuation Increase |
Knowledge Retention |
Table 1 – AI Values for the Business
Source: Adapted from Mays (apud GREENHALG, 1997).
Engineering and Artificial Intelligence
Engineering, an exact science, is based on mathematics but is not limited to it. People, processes, information are very important in day-to-day activities. Artificial Intelligence, pervasive, can act in various use cases in engineering.
In mining, AI acts in safety, for example, with computer vision for identification of the presence of safety equipment – PPEs, as well as in risk identification, production forecasting, robotics, and even in the operation of autonomous vehicles.
There are cases of checking water in wagon loads, calculating the volume of ore present in the wagons with just one camera over the rail. The prediction of maintenance of parts or equipment also reaches another level with the analysis of AI identifying the best time to stop for maintenance and refueling in order to optimize results.
The classification of materials with AI models has strong results, for example, with the analysis of material similarity. Models that use Euclidean distance or cosine, for example, can identify similar items, materials that can be used in the absence of others, or even to serve customers looking for products with close specifications. Grus (2016, p. 141) defines a model as the specification of a mathematical (or probabilistic) relationship existing between different variables and complements it with the definition of machine learning as the act of creating and using models that are learned from data (GRUS, 2016, p. 142). We can conclude that data is of utmost importance for good models to be created and to learn, generating quality results. The distances measured between the materials, therefore, depend on a good database and its characteristics.
Product Engineering
Product engineering has needs ranging from research and development to sales and production planning. In this research process, which can involve thousands of material items, human work becomes more complex and even impossible without the support of technology, systems, and now AI.
The pains of this industry include: 1) Identifying similar products 2) Creating differentiated products 3) Planning sales by SKU in all regions 4) Forecasting sales influenced by rain/climate future 5) Planning production aiming to improve indicators – OTIF (on time in full), for example.
This study on a flavor/aroma industry, working with over 250,000 items for the composition of its products, has gone through evolutions in AI solutions to improve the process of creating new flavors (R&D) through two artificial intelligences. The first was a chatbot for conducting the search for a specific flavor among the more than 200,000 items. The second was a machine learning model for material similarity, using a cosine distance algorithm.
The project of this second AI enabled extreme speed, impossible to be achieved by human knowledge, given the immense proportion of product alternatives, variables, and restrictions in the search. Not only was the creation of new products accelerated, but the search for similar items also became common and fast.
Lee et al. (2023) represent an odor map where these are plotted by the first and second principal components (PC) of their POM coordinates – Principal Odor Map with 256 dimensions. Each graph shows a subset of related odor labels, visually showing, even in two dimensions, the ability to identify similarity or not between odors. See figure 4.
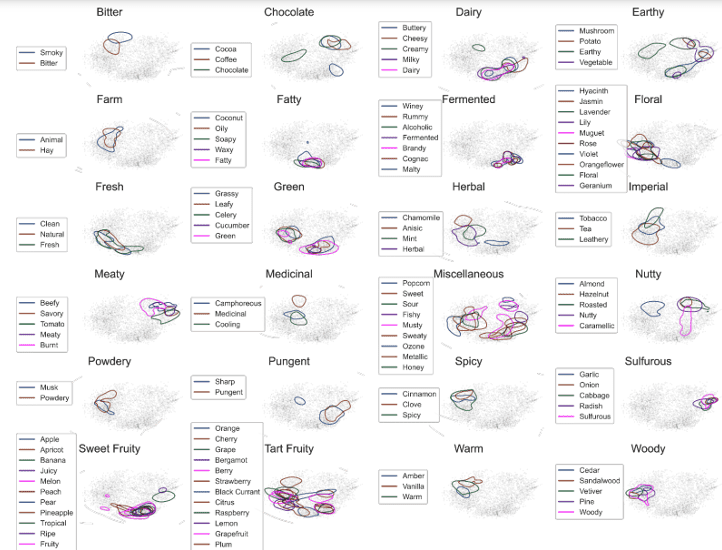
Figure 4 – Odor Map
Source: Lee et al. (2023).
Regarding sales planning, as one of the products sold was affected by climate, rainfall throughout Brazil, a regression machine learning model was developed that analyzed the historical sales series and added to the model the future climate, rain forecast. The great differential in this project lies in the AI’s ability to make a forecast for each SKU – Stock Keeping Unit for each region of Brazil. Previously, the company, given the number of existing SKUs, made sales forecasts by product family and for Brazil as a whole. In a few months, the AI project generated assertive forecasts, by region, by SKU, and with a vision of the future climate that impacts about 20% of the forecasts. Something unimaginable previously. This is the differential, the competitive advantage over the competition. The sum of the “AIs” generating results. This is one of the reasons that require maturity in AI, according to GARTNER – AI maturity adding several models in production. Each AI delivering results according to table 1 contributes in an integrated way to a differentiated level against the competition.
Final Considerations
Engineering and mining are undergoing an irreversible revolution, the incorporation of robotics and artificial intelligence that can read, understand and generate actions on a high volume of data, truly transforming the organization with a data-driven focus. Whether with IoT data or relational systems, AI models bring speed, assertiveness, and quality in decision-making in conjunction with humans. The engineers of the “future” will be curators of AI solutions in their daily life, contributing to the evolution of models, learning, and teaching the machine to perform repetitive and more complex tasks. The engineer who does not use AI will lose space to those who use it and know how to act together. Likewise, companies that do not embark on the AI journey, seeking its maturity, will lose space when competing in a “manual” way, at a total disadvantage.